ThreatFabric extends Fraud Risk Suite with advanced Behavioural Analytics
01 September 2022
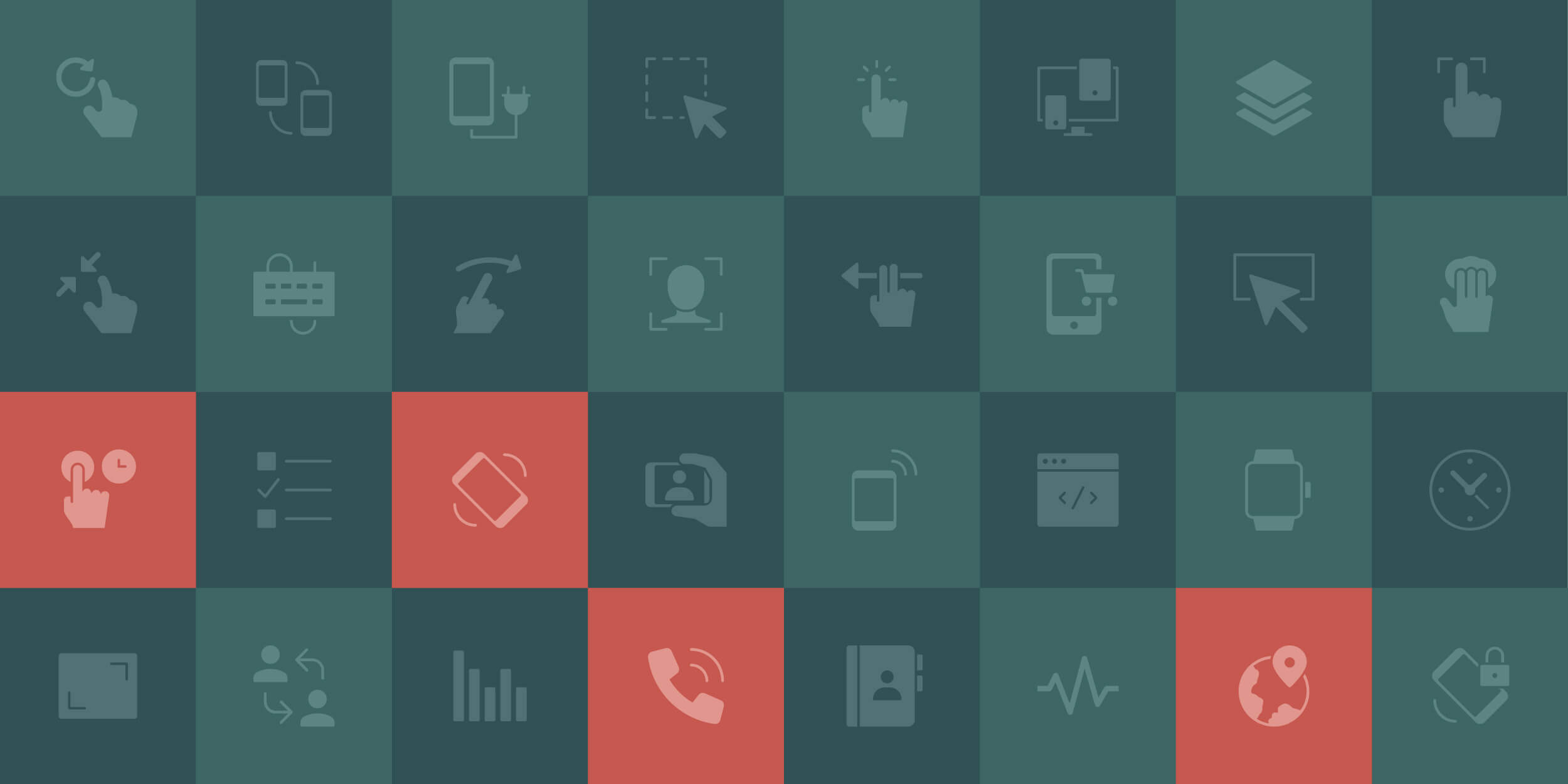
Jump to
A fully layered behavioural analytics solution to defend against the hybrid-fraud era
During the past year, ThreatFabric has closely collaborated with banks to develop layered behavioural analytics as an extension of our Fraud Risk Suite. Behavioural analytics safeguards the online customer experience against fraud, including social engineering attacks such as voice scams (Vishing).
What Is Behavioral Analytics?
Behavioural analytics can be defined as a user’s interaction with their digital devices. It combines sensor data from keyboard, swipe, and touch (gestures), which directly relate to their cognitive behaviour. An example of this could be long pauses while typing that could indicate doubt.
Behavioural analytics enables us to distinguish between legitimate customers, and cybercriminal activity. Behavioural analytics enhances digital identity proofing and removes friction by avoiding step-up authentication (MFA).
Behavioural Analytics is the Strongest Weapon Against Online Fraud by Vishing
During social engineering attacks, fraudsters entice you to perform actions on their behalf. A good example is voice phishing (Vishing). Fraudsters first create trust by spoofing the phone number (caller ID) of your bank or government agency. With coercion, they convince you to transfer money to a so-called “secure vault” to steal all your funds.
It is a myth that this type of fraud only happens to elderly or less tech-savvy victims. Fraudsters continuously exploit people in vulnerable situations. Over the past years a significant number of fraud cases have been reported affecting highly intelligent expats. Their vulnerability is their urgency to open a bank account in a new country. Without a bank account they can’t receive payments, making it harder to rent a house for example. Already experiencing the stress of adapting to a new life, these expats received phone calls from so-called bank employees. They went through what they thought were the normal procedures of opening a new account, only to later discover that they have been swindled.
Detecting social engineering attacks within customer journeys is a hard challenge. Consequently, understanding how customers interact with their devices is key. The same cognitive footprint that humans leave behind when interacting with their devices is the answer to detecting fraud by social engineering. Digital behaviour includes, but is not limited to:
- long pauses in the typing rhythm indicating doubt;
- pointless mouse movements that could indicate coercion while interacting with the online banking website;
- mobile swipes and touch sensors that show a high degree of stress.
In this way, behavioural analytics is used to continuously validate and reinforce the digital identity of a customer while performing risky actions such as payments or changing the address book.
Biometrics vs Analytics
Cybersecurity is plagued with the misuse of terminology, which creates significant confusion for legislators as well as security and privacy officers of enterprise organisations that must perform risk assessments on new technologies.
We would like to set the record straight by using the term “behaviour analytics” instead of the following terms, which, in our view, are incorrect:
- Behaviour biometrics
- Soft biometrics
In essence, behaviour analytics extracts digital behavioural patterns from humans interacting with their devices.
Let’s take “key flight” (statistical values of typing speed) as an example. Key flight data on its own is not bound to the biometrics of a person such as face, or fingerprint recognition, nor is it bound to an emotion like hesitation. With the use of AI models it is possible to use key flight data to identify slow typing patterns as behaviour anomaly.
The models are built on the historical typing rhythm of a person. These models allow our solution to perform mathematical judgments, based on a specific feature of the model, to identify fraud by social engineering. For example, slow typing could indicate that the victim is being coached to perform actions by a fraudster.
The behaviour data on its own is not strong enough to be classified as any form of biometrics. Biometric authentication controls such as Face ID or Touch ID are bound to physical aspects that a person cannot get rid of easily. In contrast, behaviour analytics is an additional digital identity proofing method. Behaviour analytics allows for continuous authentication to remove friction by avoiding step-up authentication strategies, or identify coaching fraud (Authorised Push Payments). Still, on its own, it can’t be classified as soft, very soft, nor hard biometrics.
R&D without over trusting technology and introducing BIAS
ThreatFabric was founded by RED-teamers and mThreatFabric was founded by RED-teamers and malware reverse engineers. This hacker mindset, combined with the hands-on fraud-fighting experience we gained while employed by ABN AMRO Bank, has taught us not to overestimate the effectiveness of single-point technology in guarding against ever-changing fraud vectors. We believe that there should be a healthy balance between what AI models can classify as fraudulent, and the distinctive technical footprint fraudsters leave on a victim’s device.
Considering that behavioural analytics is based on how a person interacts with their devices, we have seen that there are many scenarios that can immediately impact the classification of the AI models. Example scenarios include mild migraine, which influences cognitive behaviour, or operating system, browser and API changes. We have been actively coached by Patrick Bours, a professor in Behavioural Biometrics at Norwegian University of Science and Technology, and owner of Bours Behavioural Biometrics. His vast knowledge of behavioural analytics, together with our hacker mindset on how fraudsters abuse technology, has given us key insights into how to use this technology without overestimating its place in the fraud defence layers.
Patrick Bours: “Even though behavioural biometrics and behavioral analysis use similar techniques, the goals are completely different. Where biometrics aims to identify or authenticate a single person, is analytics used to detect anomalies and deviations in normal behavior.”
Our overall conclusion is that behavioural analytics is very effective in detecting advanced social engineering attacks, such as voice phishing, and differentiating between a genuine customer and a cybercriminal (improving digital identity proofing).
Since the reliability of this technology can be influenced by external factors (migraine, new keyboard, updates), we base the overall risk score by combining behavioural analytics with technical proof of the attacker’s footprint on the victims’ devices. The technical footprints of potential vishing include:
- the victim receiving a VOIP call while actively banking on the page or mobile app;
- the victim installing legitimate remote access support tools such as AnyDesk or TeamViewer (for the first time);
- these remote access support tools are active while they are banking.
This layered approach uses the technical footprint of attackers as hard technical proof and combines this with the customer’s behavioural analytics anomalies to expose fraud by social engineering (vishing).
Orchestrating Multiple Technologies to Defend Against Hybrid Fraud Attacks
A recent example of hybrid fraud is Telephone Oriented Attack Delivery (TOAD), in which the attacker uses vishing to lure the victims into installing an Android banking trojan. The phishing URL leading to the trojan is sent via SMS to the victim. The victim is then being called by a scammer (fake banking employee) urging them to install a “very important” security update before they are allowed to continue with their online banking.
A single-point solution that is only focused on behavioural analytics would not be able to reliably detect these type of TOAD attacks since this attack operates on two significantly different attack layers (malware and vishing), which defines a new age of fraud: hybrid!
The only reliable way to protect against such a hybrid fraud threat is by orchestrating different pillars of fraud indicators (malware and social engineering attacks) with a strong foundation of device and fraud intelligence inside an overall risk scoring engine.
Enabling frictionless MFA
Classic authentication using passwords and multi-factor authentication can be an effective defense against hackers, but it only takes place at one point in time: at the start of a banking session. It also adds friction to the customer journey. Once past the authentication point, users can do whatever they want in their “secured” environment. And so can the malware on their device or an attacker that performs voice phishing inside the entire online journey of the victim.
Once we start measuring many data points (sensors) in a device to perform behavioural analysis in real-time and continuous manner, it becomes possible to assign a continuous trust level to a customer behind a device. With this in place, a session can be stopped automatically when behavioural anomalies become too suspicious or frequent. Behavioural analytics can elevate trust without interrupting the user journey, enhancing user experience and enable your existing IAM products to perform Continuous Adaptive Trust (CAT) of the users claim, resulting in frictionless multifactor authentication (MFA).
About ThreatFabric
ThreatFabric’s Fraud Risk Suite enables safe & frictionless online customer journeys by integrating industry-leading mobile threat intel, behavioural analytics, advanced device fingerprinting and over 10.000 adaptive fraud indicators. This will give you and your customers peace of mind in an age of ever-changing fraud.